AI Support Agents: The Ultimate Guide to Automating IT, HR, and Customer Support
As enterprises face ever-increasing support volumes across IT, HR, and customer service functions, these agents have emerged as a critical solution for scaling operations without proportionally increasing headcount. They handle routine inquiries 24/7, allowing human agents to focus on complex issues requiring judgment and empathy.
According to a recent Gartner report, by 2029, agentic AI will autonomously resolve 80% of common customer service issues without human intervention. This forecast underscores the rapid advancement of AI agents and their potential to streamline customer service operations by reducing reliance on human oversight for routine inquiries.
This blog serves as the definitive guide to AI Support Agents, covering everything from technical fundamentals to implementation best practices, with links to in-depth resources throughout.
.png)
What is an AI Support Agent?
An AI Support Agent is an intelligent system that combines advanced language models, enterprise knowledge, and workflow automation capabilities to handle support requests autonomously. Unlike basic chatbots that merely navigate conversation trees or respond with static answers, true AI Support Agents can:
- Understand natural language queries with context and nuance
- Access and synthesize information from various knowledge sources
- Evaluate multiple potential solutions
- Plan and execute multi-step workflows
- Take action in enterprise systems (e.g., reset passwords, create accounts)
- Learn from interactions to continuously improve
The key distinction between AI Support Agents and previous generations of support automation tools is their ability to handle ambiguity and take autonomous action. While traditional chatbots could only respond based on pre-programmed rules, modern AI Support Agents leverage large language models (LLMs) to understand intent, context, and to generate appropriate solutions.
Fundamentally, AI Support Agents represent the shift from reactive, script-based support tools to proactive, reasoning-based autonomous systems.
Learn more about What are AI Agents →
Key Benefits of AI Support Agents
Enterprise adopters consistently report ROI within the first quarter of deployment, with the most successful implementations achieving 200-300% returns within the first year. As support volumes continue to scale exponentially across digital channels, these benefits become increasingly pronounced, creating competitive advantages for early adopters.
Implementing AI Support Agents delivers measurable improvements across several critical dimensions:
Operational Efficiency
- 70-80% deflection of routine support tickets
- 30-40% reduction in operational expenses
- 65% faster average resolution times for common issues
- 24/7 availability without staffing challenges
Enhanced Experience
- Instant responses without queues or wait times
- Consistent quality regardless of volume fluctuations
- Self-service resolution for users who prefer it
- Multilingual support without additional resources
Strategic Advantages
- Detailed analytics on common issues and knowledge gaps
- Identification of process inefficiencies and improvement opportunities
- Redeployment of human expertise to complex, high-value activities
- Scalable support operations that grow without proportional costs
These agent benefits translate to substantial ROI, with enterprises typically seeing payback periods of 3-6 months for comprehensive AI Support Agent implementations.
How AI Support Agents Work (Technically)
Understanding the technical architecture of AI Support Agents is essential for effective implementation. Modern systems typically incorporate several key components:
Foundation Models
Advanced AI Support Agents utilize large language models (LLMs) as their core reasoning engine. These models understand natural language, interpret user intent, and generate contextually appropriate responses. Enterprise-grade solutions use either fine-tuned versions of models like GPT-4 or Claude, or proprietary models specifically trained for support scenarios.
Knowledge Integration
To provide accurate, organization-specific responses, AI Support Agents must access enterprise knowledge.
- Document indexing and embedding of help center articles, FAQs, and documentation
- Processing of historical ticket data to learn from previous resolutions
- Integration with product knowledge bases and technical resources
- Continuous synchronization as knowledge bases evolve
Reasoning & Planning
What separates true AI Support Agents from simple chatbots is their reasoning capability. When presented with a request, the system:
- Evaluates the nature of the request
- Determines whether it has sufficient information
- Plans a resolution approach
- Identifies necessary steps and tools
- Executes the plan or escalates when appropriate
System Integrations
To deliver end-to-end resolution, AI Support Agents connect with enterprise systems through:
- API integrations with ticketing platforms (Zendesk, ServiceNow, Jira)
- Workflow automation tools
- User management systems
- Communication platforms (Slack, Teams)
- Custom business applications
Feedback Loop & Learning
AI Support Agents improve over time through structured feedback mechanisms:
- Resolution ratings from end-users
- Manual reviews by support specialists
- Analysis of escalation patterns
- Periodic retraining with new data
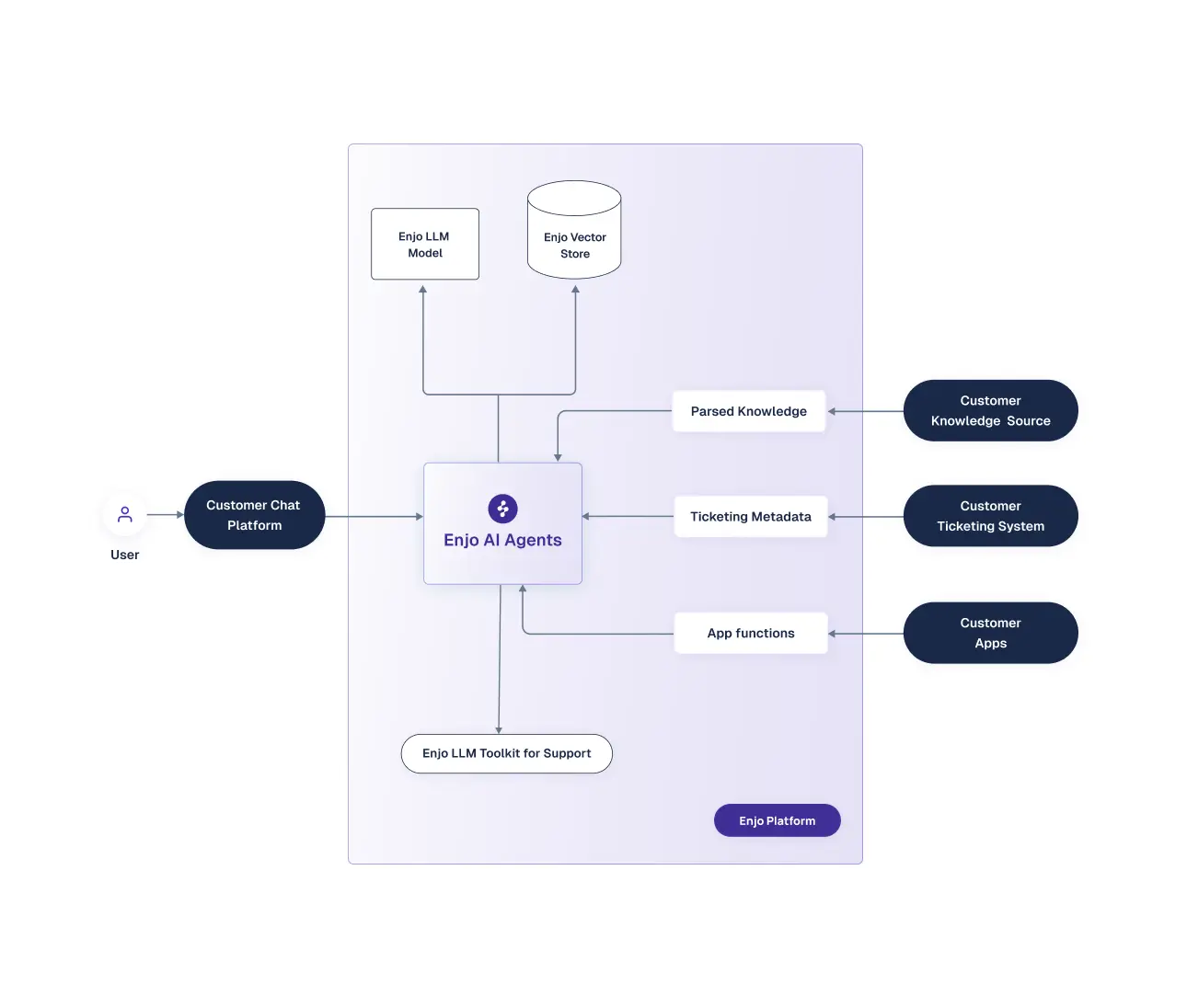
This architecture enables the autonomous handling of support requests from initial understanding through to resolution, with appropriate human oversight for quality control.
Learn more about How AI Support Agents Work: Behind the Scenes →
Primary Use Cases of Support Agents
While implementations exist in virtually every business department, three key areas have emerged as the primary adoption targets due to their combination of clear ROI potential and well-defined knowledge requirements. AI Support Agents excel across multiple support functions within the enterprise:
Customer Support
For customer-facing support functions, AI Support Agents enable:
- 24/7 first-line response to product and service inquiries
- Automatic classification and routing of complex issues
- Guided troubleshooting for common products problems
- Order status checking and transaction history retrieval
- Account management and profile updates
These capabilities result in faster resolution times, higher customer satisfaction, and reduced support costs for organizations with high-volume customer inquiries.
Learn more about AI Support Agents for Customer Service →
IT Helpdesk
Within internal IT support, AI Support Agents streamline operations by handling:
- Password resets and account unlocks
- Software access provisioning
- Basic troubleshooting for common IT issues
- Navigation assistance for enterprise applications
- System status updates and outage information
IT organizations typically see 60-70% automation rates for common helpdesk tickets, allowing skilled IT staff to focus on complex infrastructure and security priorities.
Learn more on AI Support Agents for IT Helpdesk →
HR Support
Human Resources departments leverage AI Support Agents for:
- Benefits enrollment and policy questions
- Time-off requests and approvals
- Document retrieval (pay stubs, tax forms)
- Onboarding process guidance
- Policy clarification and procedural information
These implementations reduce administrative burden on HR teams while providing employees with immediate answers to common questions.
Read more: AI Support Agents for HR →
Trends in AI Support Agents
The AI Support Agent landscape continues to evolve rapidly. Key trends defining the space in 2025 include:
Multi-Agent Systems
Rather than relying on a single AI agent, organizations are implementing specialized agents for different functions, working together as a coordinated system. These multi-agent setups include:
- Triage agents that classify and route requests
- Specialized domain agents with deep expertise in specific areas
- Orchestration agents that coordinate complex workflows
- Oversight agents that monitor performance and ensure quality.
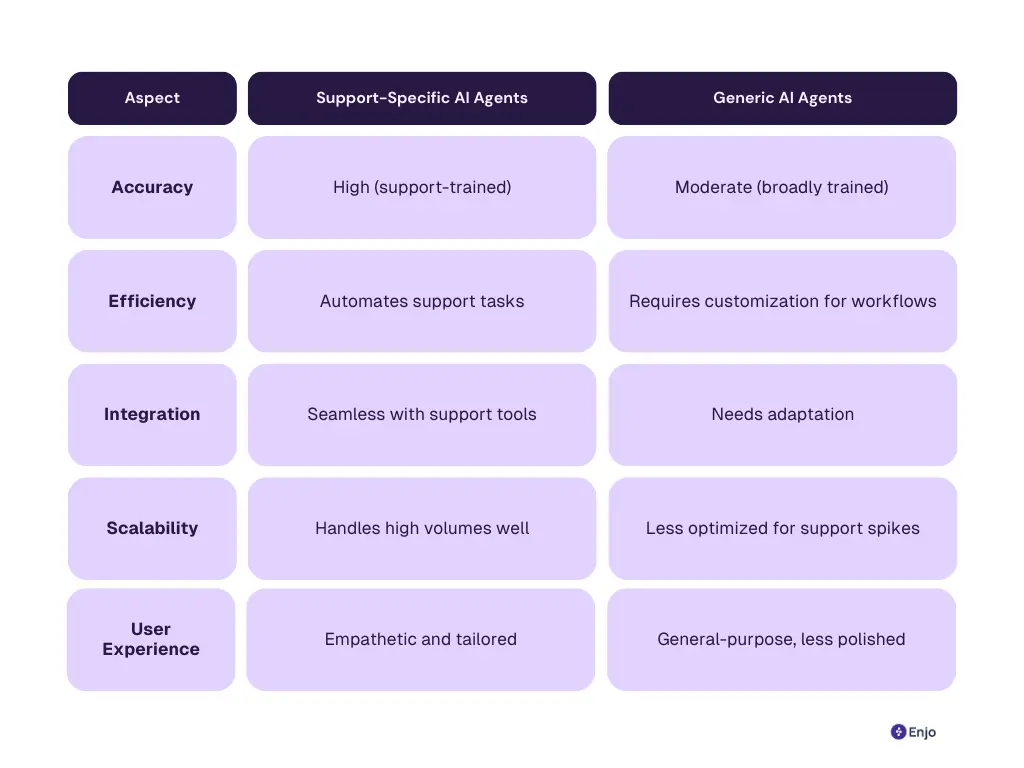
Autonomous Workflows
Advanced AI Support Agents are moving beyond conversation to execute complex, multi-step processes without human intervention. This includes:
- Cross-system operations involving multiple enterprise applications
- Decision-making based on business rules and policies
- Handling of exception cases through predefined alternatives
- Completion of entire support processes from request to resolution
Enterprise LLM Adoption
As organizations grow more sophisticated in their AI strategies, many are deploying:
- Private LLM instances with enterprise-specific training
- Domain-adapted models focused on internal terminology and processes
- Hybrid approaches combining public and private model capabilities
- Specialized models for specific business functions
Proactive Support Models
The most advanced implementations are shifting from reactive to proactive support by:
- Identifying potential issues before they affect users
- Recommending process improvements based on support data
- Delivering personalized guidance based on user behavior patterns
- Preemptively resolving anticipated problems
These trends indicate a maturing market moving from basic automation toward truly intelligent support systems that fundamentally transform enterprise operations.
Learn more about the latest AI Support Agent Trends in 2025 →
Implementation Overview
Despite the sophistication of underlying AI technology, implementation success correlates more strongly with preparation quality than with model selection or technical specifications. Successfully deploying AI Support Agents requires careful planning and execution across several key dimensions:
Data Requirements
The foundation of any effective AI Support Agent is high-quality knowledge. Organizations must prepare:
- Comprehensive documentation covering products, services, and policies
- Structured FAQ content addressing common questions
- Historical support ticket data (ideally with resolution notes)
- Process and workflow documentation for automated actions
Technical Setup
Implementation typically involves:
- Knowledge base integration and initial training
- System integrations with ticketing platforms and enterprise applications
- User interface configuration (chat, messaging platforms, email)
- Security controls and access management
- Testing environments for validation before production deployment
Rollout Strategy
Most successful implementations follow a phased approach:
- Pilot with limited scope and supervised operation
- Expansion to handle specific, well-documented use cases
- Progressive addition of more complex scenarios
- Continuous improvement based on performance data
Change Management
Ensuring adoption requires:
- Clear communication with both support teams and end-users
- Training for support specialists on working alongside AI
- Feedback mechanisms to identify and address issues
- Regular reviews and performance assessments
Organizations achieving the greatest success typically allocate 3-4 months for initial implementation and follow with continuous optimization based on usage patterns and feedback.
Learn more about how to implement AI Support Agents →
Training AI Support Agents
Effective training represents the critical differentiator between AI Support Agents that merely respond and those that autonomously resolve. While the underlying language models provide conversational capabilities, enterprise-specific knowledge determines actual resolution effectiveness. The process involves several interconnected components:
Knowledge Base Preparation
- Auditing existing documentation for accuracy and completeness
- Structuring content for optimal retrieval and relevance
- Creating documentation for common but undocumented processes
- Establishing maintenance workflows for knowledge updates
Quality Control Mechanisms
- Defining confidence thresholds for autonomous resolution
- Establishing human review processes for uncertain cases
- Creating feedback loops for continuous improvement
- Setting up monitoring for accuracy and resolution rates
Governance Framework
- Developing clear policies for appropriate AI agent use
- Establishing oversight responsibilities and audit trails
- Creating escalation paths for complex or sensitive issues
- Defining measurement standards for performance evaluation
Organizations that invest in thorough training processes typically achieve 90%+ accuracy rates for in-scope scenarios, compared to 60-70% for implementations with minimal training investment.
Read More on How to Train AI Support Agents →
Different AI Agents in the Market
The AI Support Agent market includes several notable platforms with varying capabilities:
Enterprise-Focused Solutions
- Enjo: Comprehensive platform with deep workflow automation capabilities
- Moveworks: Focused primarily on IT service management
- Forethought: Specializes in customer support automation
- IBM Watson Assistant: Enterprise-grade solution with robust integration capabilities
- Agentforce: AI-powered platform with advanced reasoning and multi-agent orchestration
- Ada: Customer service-oriented solution
Platform Extensions
- ServiceNow Virtual Agent: Integrated with the ServiceNow ecosystem
- Zendesk AI: Native capabilities within the Zendesk platform
- Salesforce Einstein: Embedded within Salesforce Service Cloud
Selection criteria should include integration capabilities, enterprise security controls, training requirements, and specific functional needs based on use cases.
Learn more about the Top 10 AI Support Agents in 2025 →
Evaluating Success
Effective evaluation combines operational metrics with financial indicators to create a complete picture of transformation progress. This comprehensive approach ensures continuous improvement while validating investment decisions against tangible results.
A proper evaluation framework should incorporate metrics across several complementary dimensions:
Volume Metrics
- Ticket deflection rate: Percentage of inquiries fully resolved by AI
- Automation rate: Proportion of total support volume handled autonomously
- Escalation rate: Percentage of AI interactions requiring human intervention
Efficiency Metrics
- Mean Time to Resolution (MTTR): Average time from request to resolution
- First Contact Resolution (FCR): Percentage of issues resolved in first interaction
- Agent productivity: Support volume handled per human agent
Quality Metrics
- Customer Satisfaction (CSAT): User ratings of AI-provided support
- Resolution accuracy: Correctness of solutions provided
- Knowledge gap identification: New issues requiring documentation
Business Impact
- Cost per ticket: Total support cost divided by volume
- Support capacity: Maximum volume manageable with current resources
- ROI: Cost savings and efficiency gains versus implementation investment
Organizations should establish baseline measurements before implementation and track trends over time, with quarterly reviews to identify optimization opportunities.
Learn more about Evaluating Support Service AI Agents: Key Metrics →
Challenges and How to Address Them
While AI Support Agents offer significant benefits, organizations should be prepared to address several common challenges:
Hallucination and Accuracy Issues
- Challenge: AI models occasionally generate plausible but incorrect responses
- Solution: Implement strict knowledge retrieval frameworks, confidence thresholds, and human review for uncertain cases
Poor Data Preparation
- Challenge: Incomplete or outdated knowledge bases lead to gaps in AI capabilities
- Solution: Invest in knowledge audits, structured content creation, and regular maintenance processes
Integration Limitations
- Challenge: Lack of API access to legacy systems limits automation potential
- Solution: Develop middleware connectors, RPA bridges, or phased migration strategies for critical systems
Change Resistance
- Challenge: Support teams may view AI as a threat rather than a tool
- Solution: Focus on augmentation rather than replacement messaging, involve agents in training, and highlight high-value work opportunities
Scope Management
- Challenge: Attempting to automate too much too quickly leads to poor performance
- Solution: Begin with well-defined, high-volume use cases and expand methodically based on success
Ethical and Privacy Concerns
- Challenge: Customer and employee data require careful handling
- Solution: Implement strict data governance, minimize sensitive data usage, and maintain transparency about AI capabilities
Organizations that proactively address these challenges achieve significantly higher success rates and faster time-to-value from their implementations.
Learn more: AI Support Agent Challenges: Common Pitfalls →
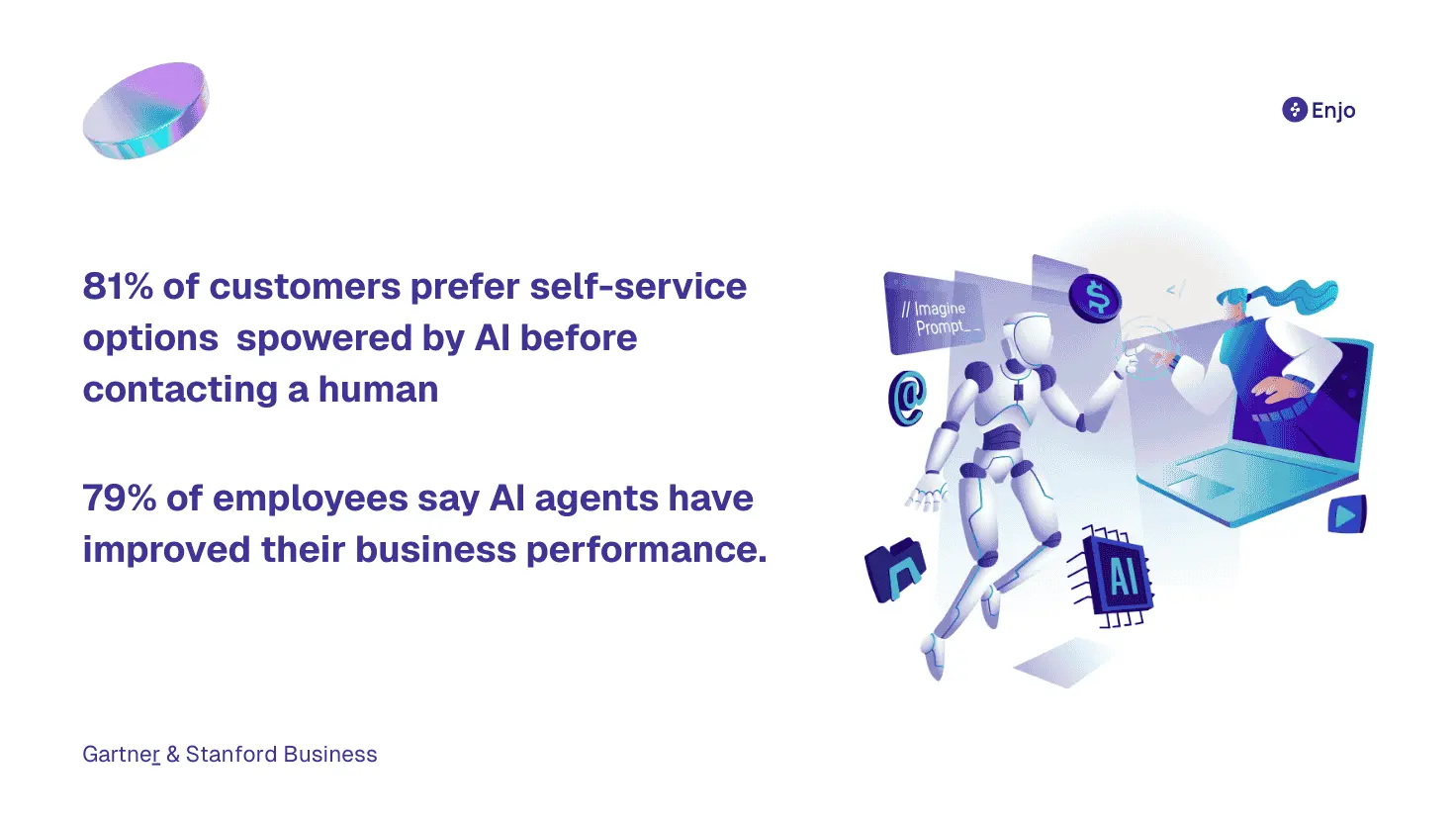
Why Enjo Stands Out
In the evolving landscape of AI Support Agents, Enjo has established itself as a leader through several distinctive capabilities:
Enterprise-Grade Adoption
- Deployed at scale by industry leaders including Netflix, Spotify, Snowflake, Aurora, DeliveryHero, and Snapchat
- Proven performance in high-volume, complex support environments
- 99.9% uptime reliability with enterprise-grade SLAs
True Autonomous Support
- Moves beyond simple Q&A to execute end-to-end resolution workflows
- Connects to enterprise systems through native integrations and APIs
- Takes action across multiple platforms to resolve issues completely
Security and Compliance
- SOC 2 compliance and enterprise-ready security controls
- Role-based access management and audit trails
- Data handling practices trusted by InfoSec teams at Fortune 500 companies
Applied AI Excellence
- Continuously refined models based on millions of enterprise support interactions
- Advanced agent architecture with specialized reasoning capabilities
- Industry-leading accuracy rates for supported use cases
These capabilities enable Enjo to deliver comprehensive support automation that scales with enterprise needs while maintaining security, reliability, and performance standards expected by IT leaders.
Conclusion
AI Support Agents represent a fundamental shift in how enterprises manage both customer and employee support functions. By automating routine inquiries and enabling autonomous resolution of common issues, these systems simultaneously reduce operational costs and improve service quality.
The technology has now matured beyond experimental applications to become a core component of enterprise support strategy, with proven implementations across industries and functions. Organizations that have successfully deployed AI Support Agents are achieving support scale that would be financially prohibitive through traditional staffing models alone.
As we move further into 2025, the capabilities of these systems will continue to expand, enabling even greater levels of autonomy and effectiveness. For enterprises facing growing support demands with constrained resources, AI Support Agents have become an essential solution rather than an optional enhancement.
To explore how AI Support Agents can transform your support operations, consider starting your free trial of Enjo.
What is an AI Support Agent?
An AI Support Agent is an intelligent system that combines advanced language models, enterprise knowledge, and workflow automation capabilities to handle support requests autonomously. Unlike basic chatbots that merely navigate conversation trees or respond with static answers, true AI Support Agents can:
- Understand natural language queries with context and nuance
- Access and synthesize information from various knowledge sources
- Evaluate multiple potential solutions
- Plan and execute multi-step workflows
- Take action in enterprise systems (e.g., reset passwords, create accounts)
- Learn from interactions to continuously improve
The key distinction between AI Support Agents and previous generations of support automation tools is their ability to handle ambiguity and take autonomous action. While traditional chatbots could only respond based on pre-programmed rules, modern AI Support Agents leverage large language models (LLMs) to understand intent, context, and to generate appropriate solutions.
Fundamentally, AI Support Agents represent the shift from reactive, script-based support tools to proactive, reasoning-based autonomous systems.
Learn more about What are AI Agents →
Key Benefits of AI Support Agents
Enterprise adopters consistently report ROI within the first quarter of deployment, with the most successful implementations achieving 200-300% returns within the first year. As support volumes continue to scale exponentially across digital channels, these benefits become increasingly pronounced, creating competitive advantages for early adopters.
Implementing AI Support Agents delivers measurable improvements across several critical dimensions:
Operational Efficiency
- 70-80% deflection of routine support tickets
- 30-40% reduction in operational expenses
- 65% faster average resolution times for common issues
- 24/7 availability without staffing challenges
Enhanced Experience
- Instant responses without queues or wait times
- Consistent quality regardless of volume fluctuations
- Self-service resolution for users who prefer it
- Multilingual support without additional resources
Strategic Advantages
- Detailed analytics on common issues and knowledge gaps
- Identification of process inefficiencies and improvement opportunities
- Redeployment of human expertise to complex, high-value activities
- Scalable support operations that grow without proportional costs
These agent benefits translate to substantial ROI, with enterprises typically seeing payback periods of 3-6 months for comprehensive AI Support Agent implementations.
How AI Support Agents Work (Technically)
Understanding the technical architecture of AI Support Agents is essential for effective implementation. Modern systems typically incorporate several key components:
Foundation Models
Advanced AI Support Agents utilize large language models (LLMs) as their core reasoning engine. These models understand natural language, interpret user intent, and generate contextually appropriate responses. Enterprise-grade solutions use either fine-tuned versions of models like GPT-4 or Claude, or proprietary models specifically trained for support scenarios.
Knowledge Integration
To provide accurate, organization-specific responses, AI Support Agents must access enterprise knowledge.
- Document indexing and embedding of help center articles, FAQs, and documentation
- Processing of historical ticket data to learn from previous resolutions
- Integration with product knowledge bases and technical resources
- Continuous synchronization as knowledge bases evolve
Reasoning & Planning
What separates true AI Support Agents from simple chatbots is their reasoning capability. When presented with a request, the system:
- Evaluates the nature of the request
- Determines whether it has sufficient information
- Plans a resolution approach
- Identifies necessary steps and tools
- Executes the plan or escalates when appropriate
System Integrations
To deliver end-to-end resolution, AI Support Agents connect with enterprise systems through:
- API integrations with ticketing platforms (Zendesk, ServiceNow, Jira)
- Workflow automation tools
- User management systems
- Communication platforms (Slack, Teams)
- Custom business applications
Feedback Loop & Learning
AI Support Agents improve over time through structured feedback mechanisms:
- Resolution ratings from end-users
- Manual reviews by support specialists
- Analysis of escalation patterns
- Periodic retraining with new data
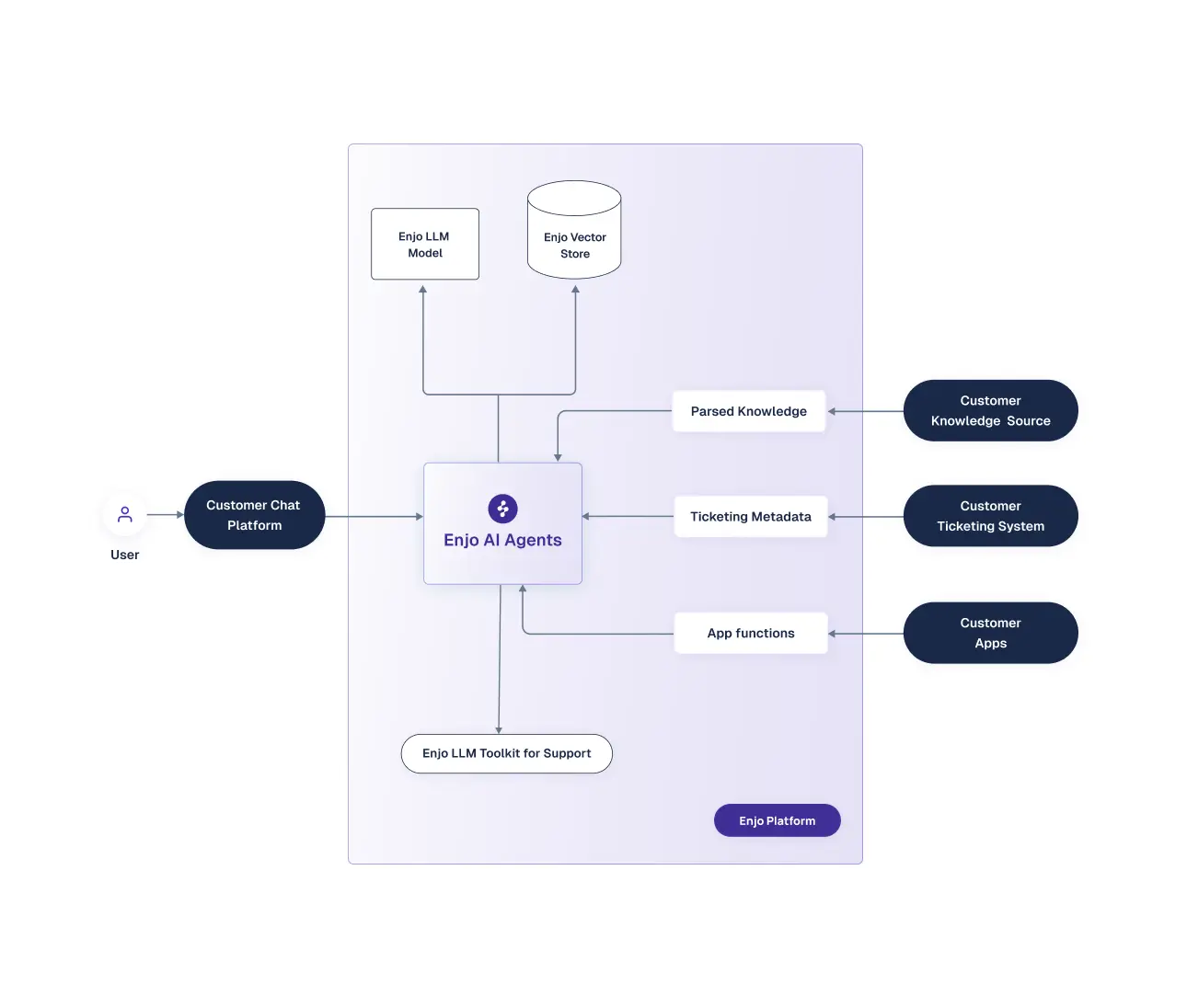
This architecture enables the autonomous handling of support requests from initial understanding through to resolution, with appropriate human oversight for quality control.
Learn more about How AI Support Agents Work: Behind the Scenes →
Primary Use Cases of Support Agents
While implementations exist in virtually every business department, three key areas have emerged as the primary adoption targets due to their combination of clear ROI potential and well-defined knowledge requirements. AI Support Agents excel across multiple support functions within the enterprise:
Customer Support
For customer-facing support functions, AI Support Agents enable:
- 24/7 first-line response to product and service inquiries
- Automatic classification and routing of complex issues
- Guided troubleshooting for common products problems
- Order status checking and transaction history retrieval
- Account management and profile updates
These capabilities result in faster resolution times, higher customer satisfaction, and reduced support costs for organizations with high-volume customer inquiries.
Learn more about AI Support Agents for Customer Service →
IT Helpdesk
Within internal IT support, AI Support Agents streamline operations by handling:
- Password resets and account unlocks
- Software access provisioning
- Basic troubleshooting for common IT issues
- Navigation assistance for enterprise applications
- System status updates and outage information
IT organizations typically see 60-70% automation rates for common helpdesk tickets, allowing skilled IT staff to focus on complex infrastructure and security priorities.
Learn more on AI Support Agents for IT Helpdesk →
HR Support
Human Resources departments leverage AI Support Agents for:
- Benefits enrollment and policy questions
- Time-off requests and approvals
- Document retrieval (pay stubs, tax forms)
- Onboarding process guidance
- Policy clarification and procedural information
These implementations reduce administrative burden on HR teams while providing employees with immediate answers to common questions.
Read more: AI Support Agents for HR →
Trends in AI Support Agents
The AI Support Agent landscape continues to evolve rapidly. Key trends defining the space in 2025 include:
Multi-Agent Systems
Rather than relying on a single AI agent, organizations are implementing specialized agents for different functions, working together as a coordinated system. These multi-agent setups include:
- Triage agents that classify and route requests
- Specialized domain agents with deep expertise in specific areas
- Orchestration agents that coordinate complex workflows
- Oversight agents that monitor performance and ensure quality.
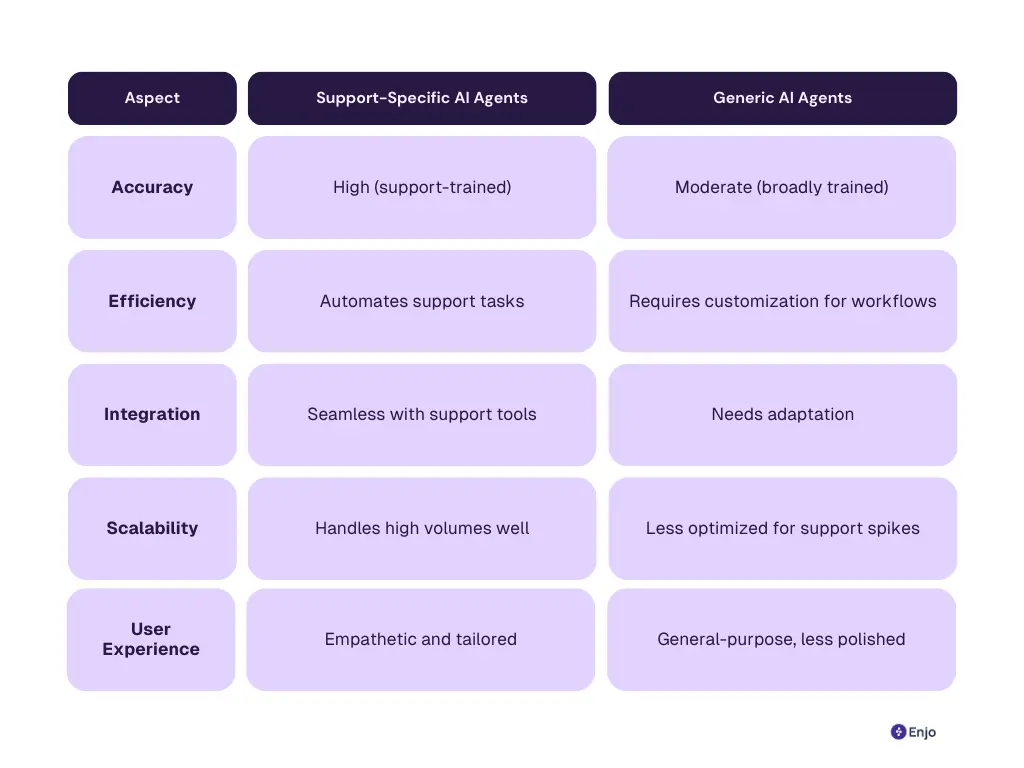
Autonomous Workflows
Advanced AI Support Agents are moving beyond conversation to execute complex, multi-step processes without human intervention. This includes:
- Cross-system operations involving multiple enterprise applications
- Decision-making based on business rules and policies
- Handling of exception cases through predefined alternatives
- Completion of entire support processes from request to resolution
Enterprise LLM Adoption
As organizations grow more sophisticated in their AI strategies, many are deploying:
- Private LLM instances with enterprise-specific training
- Domain-adapted models focused on internal terminology and processes
- Hybrid approaches combining public and private model capabilities
- Specialized models for specific business functions
Proactive Support Models
The most advanced implementations are shifting from reactive to proactive support by:
- Identifying potential issues before they affect users
- Recommending process improvements based on support data
- Delivering personalized guidance based on user behavior patterns
- Preemptively resolving anticipated problems
These trends indicate a maturing market moving from basic automation toward truly intelligent support systems that fundamentally transform enterprise operations.
Learn more about the latest AI Support Agent Trends in 2025 →
Implementation Overview
Despite the sophistication of underlying AI technology, implementation success correlates more strongly with preparation quality than with model selection or technical specifications. Successfully deploying AI Support Agents requires careful planning and execution across several key dimensions:
Data Requirements
The foundation of any effective AI Support Agent is high-quality knowledge. Organizations must prepare:
- Comprehensive documentation covering products, services, and policies
- Structured FAQ content addressing common questions
- Historical support ticket data (ideally with resolution notes)
- Process and workflow documentation for automated actions
Technical Setup
Implementation typically involves:
- Knowledge base integration and initial training
- System integrations with ticketing platforms and enterprise applications
- User interface configuration (chat, messaging platforms, email)
- Security controls and access management
- Testing environments for validation before production deployment
Rollout Strategy
Most successful implementations follow a phased approach:
- Pilot with limited scope and supervised operation
- Expansion to handle specific, well-documented use cases
- Progressive addition of more complex scenarios
- Continuous improvement based on performance data
Change Management
Ensuring adoption requires:
- Clear communication with both support teams and end-users
- Training for support specialists on working alongside AI
- Feedback mechanisms to identify and address issues
- Regular reviews and performance assessments
Organizations achieving the greatest success typically allocate 3-4 months for initial implementation and follow with continuous optimization based on usage patterns and feedback.
Learn more about how to implement AI Support Agents →
Training AI Support Agents
Effective training represents the critical differentiator between AI Support Agents that merely respond and those that autonomously resolve. While the underlying language models provide conversational capabilities, enterprise-specific knowledge determines actual resolution effectiveness. The process involves several interconnected components:
Knowledge Base Preparation
- Auditing existing documentation for accuracy and completeness
- Structuring content for optimal retrieval and relevance
- Creating documentation for common but undocumented processes
- Establishing maintenance workflows for knowledge updates
Quality Control Mechanisms
- Defining confidence thresholds for autonomous resolution
- Establishing human review processes for uncertain cases
- Creating feedback loops for continuous improvement
- Setting up monitoring for accuracy and resolution rates
Governance Framework
- Developing clear policies for appropriate AI agent use
- Establishing oversight responsibilities and audit trails
- Creating escalation paths for complex or sensitive issues
- Defining measurement standards for performance evaluation
Organizations that invest in thorough training processes typically achieve 90%+ accuracy rates for in-scope scenarios, compared to 60-70% for implementations with minimal training investment.
Read More on How to Train AI Support Agents →
Different AI Agents in the Market
The AI Support Agent market includes several notable platforms with varying capabilities:
Enterprise-Focused Solutions
- Enjo: Comprehensive platform with deep workflow automation capabilities
- Moveworks: Focused primarily on IT service management
- Forethought: Specializes in customer support automation
- IBM Watson Assistant: Enterprise-grade solution with robust integration capabilities
- Agentforce: AI-powered platform with advanced reasoning and multi-agent orchestration
- Ada: Customer service-oriented solution
Platform Extensions
- ServiceNow Virtual Agent: Integrated with the ServiceNow ecosystem
- Zendesk AI: Native capabilities within the Zendesk platform
- Salesforce Einstein: Embedded within Salesforce Service Cloud
Selection criteria should include integration capabilities, enterprise security controls, training requirements, and specific functional needs based on use cases.
Learn more about the Top 10 AI Support Agents in 2025 →
Evaluating Success
Effective evaluation combines operational metrics with financial indicators to create a complete picture of transformation progress. This comprehensive approach ensures continuous improvement while validating investment decisions against tangible results.
A proper evaluation framework should incorporate metrics across several complementary dimensions:
Volume Metrics
- Ticket deflection rate: Percentage of inquiries fully resolved by AI
- Automation rate: Proportion of total support volume handled autonomously
- Escalation rate: Percentage of AI interactions requiring human intervention
Efficiency Metrics
- Mean Time to Resolution (MTTR): Average time from request to resolution
- First Contact Resolution (FCR): Percentage of issues resolved in first interaction
- Agent productivity: Support volume handled per human agent
Quality Metrics
- Customer Satisfaction (CSAT): User ratings of AI-provided support
- Resolution accuracy: Correctness of solutions provided
- Knowledge gap identification: New issues requiring documentation
Business Impact
- Cost per ticket: Total support cost divided by volume
- Support capacity: Maximum volume manageable with current resources
- ROI: Cost savings and efficiency gains versus implementation investment
Organizations should establish baseline measurements before implementation and track trends over time, with quarterly reviews to identify optimization opportunities.
Learn more about Evaluating Support Service AI Agents: Key Metrics →
Challenges and How to Address Them
While AI Support Agents offer significant benefits, organizations should be prepared to address several common challenges:
Hallucination and Accuracy Issues
- Challenge: AI models occasionally generate plausible but incorrect responses
- Solution: Implement strict knowledge retrieval frameworks, confidence thresholds, and human review for uncertain cases
Poor Data Preparation
- Challenge: Incomplete or outdated knowledge bases lead to gaps in AI capabilities
- Solution: Invest in knowledge audits, structured content creation, and regular maintenance processes
Integration Limitations
- Challenge: Lack of API access to legacy systems limits automation potential
- Solution: Develop middleware connectors, RPA bridges, or phased migration strategies for critical systems
Change Resistance
- Challenge: Support teams may view AI as a threat rather than a tool
- Solution: Focus on augmentation rather than replacement messaging, involve agents in training, and highlight high-value work opportunities
Scope Management
- Challenge: Attempting to automate too much too quickly leads to poor performance
- Solution: Begin with well-defined, high-volume use cases and expand methodically based on success
Ethical and Privacy Concerns
- Challenge: Customer and employee data require careful handling
- Solution: Implement strict data governance, minimize sensitive data usage, and maintain transparency about AI capabilities
Organizations that proactively address these challenges achieve significantly higher success rates and faster time-to-value from their implementations.
Learn more: AI Support Agent Challenges: Common Pitfalls →
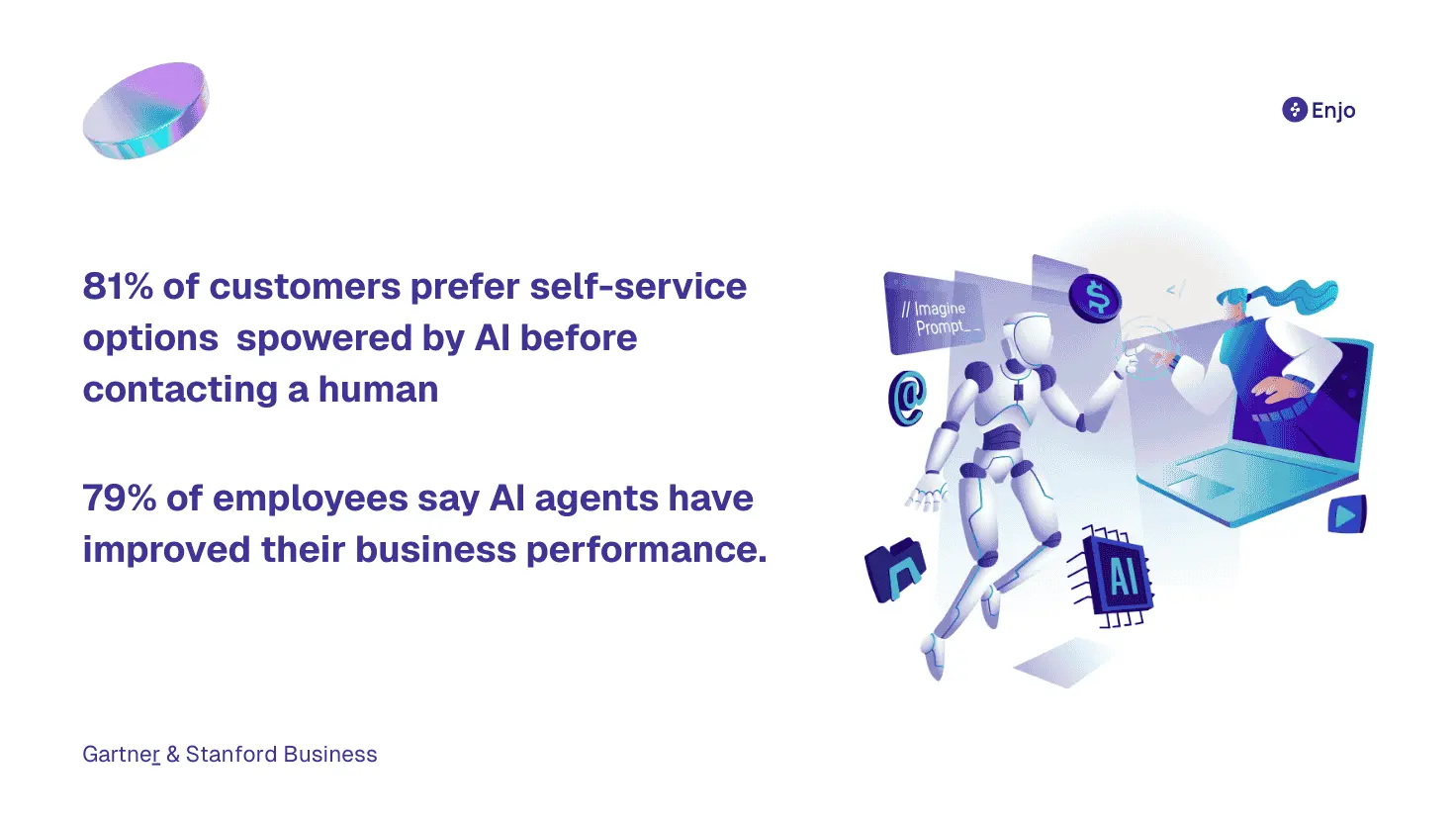
Why Enjo Stands Out
In the evolving landscape of AI Support Agents, Enjo has established itself as a leader through several distinctive capabilities:
Enterprise-Grade Adoption
- Deployed at scale by industry leaders including Netflix, Spotify, Snowflake, Aurora, DeliveryHero, and Snapchat
- Proven performance in high-volume, complex support environments
- 99.9% uptime reliability with enterprise-grade SLAs
True Autonomous Support
- Moves beyond simple Q&A to execute end-to-end resolution workflows
- Connects to enterprise systems through native integrations and APIs
- Takes action across multiple platforms to resolve issues completely
Security and Compliance
- SOC 2 compliance and enterprise-ready security controls
- Role-based access management and audit trails
- Data handling practices trusted by InfoSec teams at Fortune 500 companies
Applied AI Excellence
- Continuously refined models based on millions of enterprise support interactions
- Advanced agent architecture with specialized reasoning capabilities
- Industry-leading accuracy rates for supported use cases
These capabilities enable Enjo to deliver comprehensive support automation that scales with enterprise needs while maintaining security, reliability, and performance standards expected by IT leaders.
Conclusion
AI Support Agents represent a fundamental shift in how enterprises manage both customer and employee support functions. By automating routine inquiries and enabling autonomous resolution of common issues, these systems simultaneously reduce operational costs and improve service quality.
The technology has now matured beyond experimental applications to become a core component of enterprise support strategy, with proven implementations across industries and functions. Organizations that have successfully deployed AI Support Agents are achieving support scale that would be financially prohibitive through traditional staffing models alone.
As we move further into 2025, the capabilities of these systems will continue to expand, enabling even greater levels of autonomy and effectiveness. For enterprises facing growing support demands with constrained resources, AI Support Agents have become an essential solution rather than an optional enhancement.
To explore how AI Support Agents can transform your support operations, consider starting your free trial of Enjo.
