AI Support Agents: A Guide to Automated Support
We have all wished for our support team to handle repetitive inquiries instantly, without draining valuable human resources. AI Support Agent that delivers round-the-clock service at scale—no more busy phone lines, delayed emails, or overflowing support queues. Maintaining a exceptional service quality is no more a hurdle.
_converted.webp)
What Is an AI Support Agent?
An AI Support Agent is an intelligent system designed to interpret user queries in real time, pulling from a range of company-specific knowledge sources such as documentation, past ticket logs, and FAQs. Unlike basic chatbots that rely on rigid scripts, AI Support Agents are self-learning, meaning they continually improve with each interaction. By removing the manual workload of repetitive tasks, they allow your human agents to focus on higher-value responsibilities, ultimately enhancing the experience for both employees and customers.
Why Automated Support Matters
Human-driven support models often get bogged down with simple, repetitive requests, leading to unnecessary backlogs and sluggish response times. AI-powered customer service transforms this landscape by delivering near-instant resolutions for routine tasks—freeing up employees to tackle more complex, strategic challenges. In today’s fast-paced environment, an automated support framework is not just a tech upgrade; it’s a competitive necessity.
By investing in AI Support Agents, your organization gains a critical edge—24/7 availability, scalable operations, and consistent accuracy in handling requests. Up next, we’ll examine how these AI-driven systems seamlessly integrate into enterprise workflows, complementing and enhancing your existing support processes.
The Role of AI Support Agents in Workflows
Modern organizations juggle a constant stream of inquiries—ranging from common IT issues to complex product questions. An AI Support Agent can streamline these workflows, delivering instant, accurate answers right where your employees or customers need them. By automating repetitive tasks, you can preserve human effort for high-impact activities, reducing both costs and resolution times.
AI Customer Service vs. Traditional Support Models
Traditional support relies on human-driven responses for every ticket, sometimes leading to long wait times and inconsistent answers. In contrast, AI customer service capabilities allow an AI Support Agent to work around the clock. This uninterrupted availability empowers teams to handle a larger ticket volume without burning out staff.
Key differences include:
- Speed: AI responds immediately, while human support often has queue-based delays.
- Scalability: AI Support Agents ramp quickly to handle spikes in demand.
- Consistency: AI reduces human error and ensures uniform service quality.
Benefits of Deploying AI Support Agents
Reduced Operational Costs
Every support request handled by AI translates to lower staffing expenses and minimized employee workload. Over time, these savings add up substantially.
Faster Resolution Times
An AI Support Agent can resolve simple issues almost instantly, freeing skilled human agents to tackle more intricate inquiries. This leads to happier end users and a more efficient service desk.
Better User Experience
Consistency is king in support. AI systems maintain a standardized support approach, delivering reliable solutions every time. Users also benefit from quicker turnaround and 24/7 availability.
Data-Driven Insights
Many AI Support Agents provide analytics on ticket volume, response accuracy, and trending issues. These insights enable us to spot problems early and proactively refine their knowledge bases.
How AI Support Agents Function
An AI Support Agent works by understanding the context of each query and applying advanced Machine Learning and Natural Language Processing (NLP) to generate accurate, on-demand responses. Rather than sticking to rigid scripts, these agents learn from user interactions and existing company data, delivering consistent, real-time customer service. Below, we’ll break down the core technologies and steps behind AI-powered support.
Key Technologies: NLP and Machine Learning
Natural Language Processing (NLP):
- Enables an AI Support Agent to interpret user questions, detect intent, and respond conversationally.
- Identifies key phrases and topics in real-world language, even when users don’t phrase queries perfectly.
Machine Learning:
- Learns from historical data—like past tickets, FAQs, or documentation—and continuously refines responses.
- Allows the agent to draw connections between issues and solutions, improving accuracy with each interaction.
Combining NLP and Machine Learning makes an AI Support Agent resilient and adaptive, capable of understanding new or unexpected user requests over time.

Integrations with Knowledge Bases and Ticketing Systems
For maximum efficiency, an AI Support Agent connects directly to your organization’s ticketing platform—such as Jira, Salesforce Cases, Zendesk, or ServiceNow. This integration streamlines the process of creating, updating, or escalating support requests. Additionally, linking the agent to your knowledge base (product guides, FAQs, manuals) ensures it can swiftly retrieve relevant information.
CTA (Mid-Content): Want to see how seamless these integrations can be? Create your first AI Service Desk Agent with Enjo and unify your support
Continuous Learning and Improvement
A true hallmark of a modern AI Support Agent is the ability to continuously learn. Each interaction—successful or not—feeds back into a training loop, refining the agent’s language models. Over time, your AI solution transforms into a robust, context-aware resource, capable of handling increasingly complex tickets while maintaining quality responses.
- Feedback Loops: Solicits user or agent ratings to gauge response helpfulness.
- Incremental Updates: Incorporates new product releases, policies, or FAQs into its knowledge repository automatically.
By systematically analyzing ticket outcomes and user satisfaction metrics, the AI Support Agent can identify and close knowledge gaps, ultimately boosting efficiency and user confidence in the system.
Generations of AI Support Tools
The concept of automating support has evolved significantly over time. Understanding these generations helps illustrate where modern platforms—like Enjo—fit in the broader landscape of AI customer service solutions.
Gen 1: Rule-Based Chatbots
- Rigid Script Flows
Early chatbots adhered strictly to predefined scripts and if-this-then-that rules. Any query outside their programming would confuse them. - Limited Scope
These solutions could handle only basic tasks—like providing office hours or simple FAQs—due to their lack of true language understanding. - High Maintenance
Updates required manual intervention. Admins often had to rewrite entire decision trees when new support scenarios emerged.
Gen 2: Machine Learning Chatbots
- Data-Driven Responses
With machine learning, chatbots could understand a wider range of inputs by analyzing existing ticket data or FAQs. - Improved Accuracy
Classification models and intent recognition algorithms improved response quality. However, they still struggled with complex or multi-part inquiries. - Labor-Intensive Training
While more flexible than rule-based systems, Gen 2 chatbots needed large, labeled datasets and frequent tuning to maintain high performance.
Gen 3: Generative AI Agents
- Advanced Language Models
Generative AI leverages robust NLP frameworks, enabling the agent to understand nuance and context in user queries. - Dynamic Learning
A Gen 3 AI Support Agent can explore multiple knowledge sources—like historical tickets or product documentation—to craft real-time, context-aware answers. - Proactive & Autonomous
These agents don’t just respond; they can initiate actions, such as creating tickets or updating workflows, reducing your team’s manual workload.
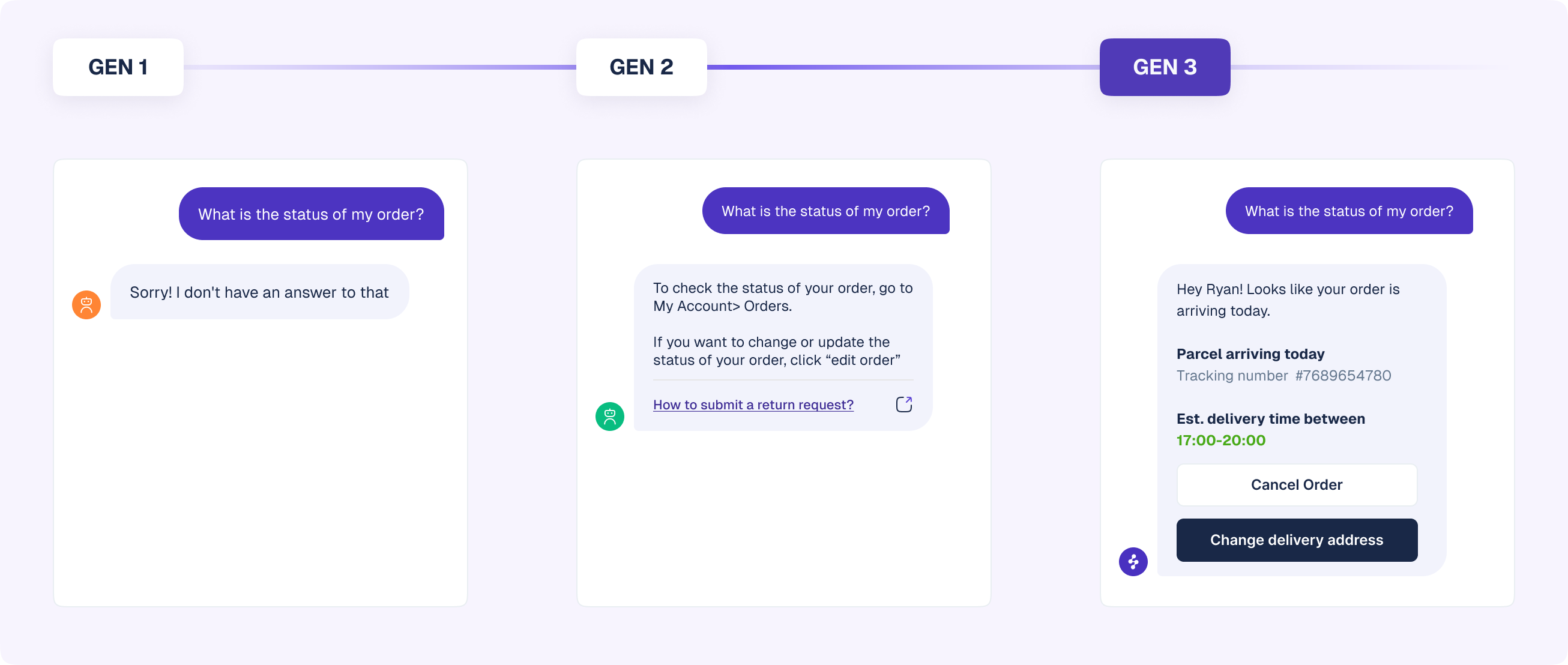
Compared to Gen 1 and Gen 2, the Gen 3 AI Support Agent stands out for its adaptability, efficiency, and deeper conversational intelligence. By adopting a solution at this level, enterprises can drastically streamline day-to-day support, enhance user satisfaction, and future-proof their service model.
Key Considerations for Implementing AI-Powered Customer Service
Before rolling out an AI Support Agent across your organisation, it’s essential to address a few foundational elements. By planning for data accuracy, security, seamless integrations, and ongoing optimization, you set your AI agents up for success from day one.
Data Preparation and Governance
An AI Support Agent relies on clean, well-structured data to generate accurate responses. This means:
- Consolidating FAQ Content and Past Tickets: Gather the most common questions, resolutions, and product documentation in a central knowledge base.
- Ensuring Data Quality: Clean up outdated entries, remove duplications, and confirm that all information is up to date.
- Implementing Access Controls: Regulate who can update or delete key articles, preventing version conflicts and misinformation.
Security and Compliance Requirements
Especially for mid to large enterprises, security and compliance are non-negotiable:
- Encryption: Ensure data is encrypted both in transit and at rest.
- Role-Based Permissions: Assign user privileges carefully so that only authorized team members can access sensitive data.
- Regulatory Standards: Verify that the AI platform meets relevant regulations (e.g., GDPR, HIPAA, SOC 2) depending on your industry.
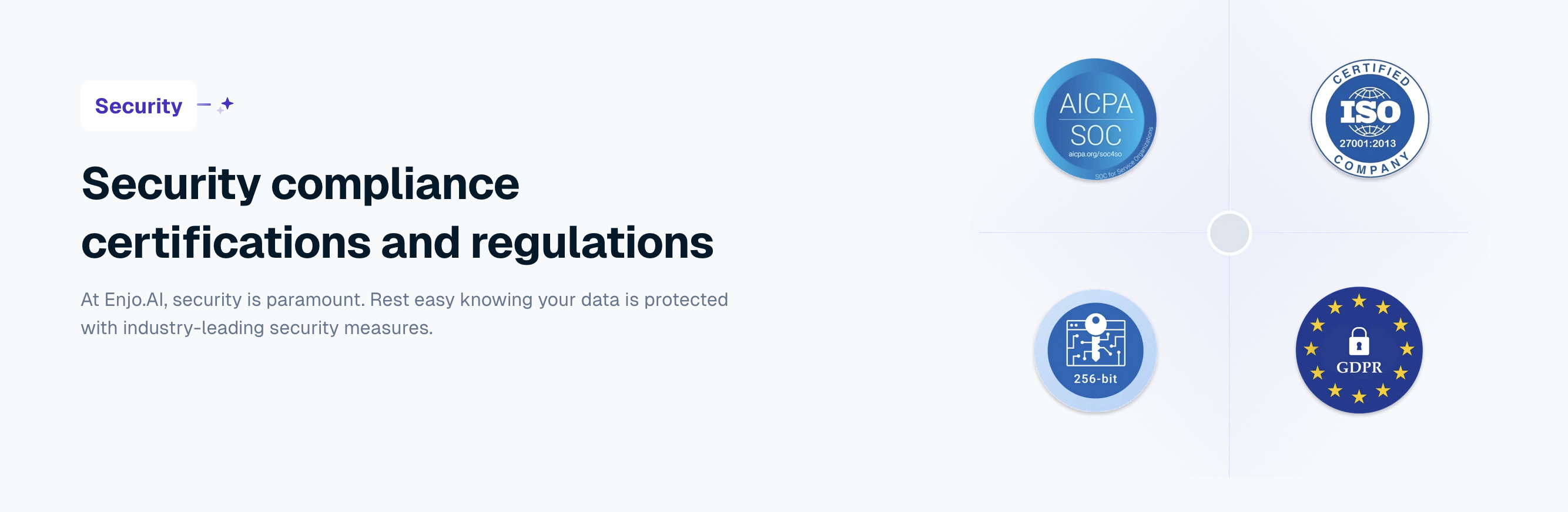
Integration Strategy with Existing Workflows
An AI Support Agent becomes far more powerful when it connects seamlessly to your existing tech stack:
- Ticketing Systems: Integrate with Jira, Salesforce Cases, Zendesk, or ServiceNow for creating and managing requests.
- Collaboration Channels: Deploy the agent in Slack or Microsoft Teams so users can chat and resolve issues in real time.
- Knowledge Repositories: Link to Confluence, SharePoint, or other document hubs where relevant content resides.
Monitoring, Analytics, and Continuous Optimization
Implementing an AI Support Agent is not a “set it and forget it” scenario. Regular evaluations and iterative improvements are crucial:
- Performance Dashboards: Track metrics like average resolution time, ticket deflection rate, and user satisfaction.
- Feedback Mechanisms: Allow users and support staff to rate the helpfulness of AI-generated responses.
- Ongoing Training: Update agent knowledge with new product information or policy changes to keep support accurate and up to date.
Top Stats and Facts About AI Support Agents
Industry Benchmarks and ROI Indicators
- Ticket Deflection Rates: Studies indicate that intelligent AI agents can deflect up to 70-80% of low-level support tickets, freeing human agents to focus on higher-complexity tasks.
- Cost Savings: By automating routine queries, enterprises often see a 30-40% reduction in operational expenses—savings that can be reinvested in strategic initiatives.
- User Satisfaction: Faster response times lead to improved CSAT scores. Organizations report boosts ranging from 10-20% after integrating AI-driven support.
- Employee Productivity: Internal teams leveraging an AI Support Agent experience less administrative burden, enabling them to handle complex issues or focus on value-added projects.
Adoption Rates and Future Projections
- Growing Demand: Gartner predicts that by 2026, almost 75% of customer service interactions will be handled by AI-driven tools.
- Industry-Wide Shift: Sectors like IT, e-commerce, and customer support operations see particularly rapid AI adoption—fueled by rising customer expectations and a need for round-the-clock service.
- Continuous Evolution: As AI language models grow more sophisticated, future AI Support Agents will begin to predict user needs before they arise, offering truly proactive and personalized services.
The Evolving Role of AI in Enterprise
The AI Support Agent is no longer a futuristic vision—it’s a practical reality reshaping support and overall workflows. Where early AI solutions focused on handling predefined tasks, today’s generative AI tools go beyond reactive support to proactively anticipate user needs.
From Reactive Support to Proactive Solutions
Traditional AI implementations responded solely to user prompts. Modern AI agents harness deep learning models, enabling them to:
- Predict User Queries: Anticipate next steps based on historical patterns.
- Preempt System Failures: Flag potential technical issues or policy gaps before they escalate.
- Offer Customized Recommendations: Tailor responses based on individual user profiles or known preferences.
By shifting to proactive engagement, the AI Support Agent helps users solve problems they might not even realize they have—ultimately boosting efficiency across the organisation.
How AI Shapes the Future of Work
Today’s workforce demands rapid communication and immediate access to resources. AI-driven solutions address these needs by:
- Streamlining Collaboration: Integrating with tools like Slack or Teams to provide on-demand insights without leaving the conversation.
- Driving Operational Excellence: Automating mundane tasks, so teams can allocate their time to strategic endeavors.
- Enhancing Knowledge Management: Continuously learning from every interaction, reducing knowledge silos across departments.
As AI evolves, we can expect new capabilities, including sentiment analysis for more empathetic responses and advanced predictive analytics for preemptive troubleshooting. By staying ahead of these trends, organizations can transform their operations, employee engagement, and customer satisfaction with a reliable AI Support Agent at the core.

What Is an AI Support Agent?
An AI Support Agent is an intelligent system designed to interpret user queries in real time, pulling from a range of company-specific knowledge sources such as documentation, past ticket logs, and FAQs. Unlike basic chatbots that rely on rigid scripts, AI Support Agents are self-learning, meaning they continually improve with each interaction. By removing the manual workload of repetitive tasks, they allow your human agents to focus on higher-value responsibilities, ultimately enhancing the experience for both employees and customers.
Why Automated Support Matters
Human-driven support models often get bogged down with simple, repetitive requests, leading to unnecessary backlogs and sluggish response times. AI-powered customer service transforms this landscape by delivering near-instant resolutions for routine tasks—freeing up employees to tackle more complex, strategic challenges. In today’s fast-paced environment, an automated support framework is not just a tech upgrade; it’s a competitive necessity.
By investing in AI Support Agents, your organization gains a critical edge—24/7 availability, scalable operations, and consistent accuracy in handling requests. Up next, we’ll examine how these AI-driven systems seamlessly integrate into enterprise workflows, complementing and enhancing your existing support processes.
The Role of AI Support Agents in Workflows
Modern organizations juggle a constant stream of inquiries—ranging from common IT issues to complex product questions. An AI Support Agent can streamline these workflows, delivering instant, accurate answers right where your employees or customers need them. By automating repetitive tasks, you can preserve human effort for high-impact activities, reducing both costs and resolution times.
AI Customer Service vs. Traditional Support Models
Traditional support relies on human-driven responses for every ticket, sometimes leading to long wait times and inconsistent answers. In contrast, AI customer service capabilities allow an AI Support Agent to work around the clock. This uninterrupted availability empowers teams to handle a larger ticket volume without burning out staff.
Key differences include:
- Speed: AI responds immediately, while human support often has queue-based delays.
- Scalability: AI Support Agents ramp quickly to handle spikes in demand.
- Consistency: AI reduces human error and ensures uniform service quality.
Benefits of Deploying AI Support Agents
Reduced Operational Costs
Every support request handled by AI translates to lower staffing expenses and minimized employee workload. Over time, these savings add up substantially.
Faster Resolution Times
An AI Support Agent can resolve simple issues almost instantly, freeing skilled human agents to tackle more intricate inquiries. This leads to happier end users and a more efficient service desk.
Better User Experience
Consistency is king in support. AI systems maintain a standardized support approach, delivering reliable solutions every time. Users also benefit from quicker turnaround and 24/7 availability.
Data-Driven Insights
Many AI Support Agents provide analytics on ticket volume, response accuracy, and trending issues. These insights enable us to spot problems early and proactively refine their knowledge bases.
How AI Support Agents Function
An AI Support Agent works by understanding the context of each query and applying advanced Machine Learning and Natural Language Processing (NLP) to generate accurate, on-demand responses. Rather than sticking to rigid scripts, these agents learn from user interactions and existing company data, delivering consistent, real-time customer service. Below, we’ll break down the core technologies and steps behind AI-powered support.
Key Technologies: NLP and Machine Learning
Natural Language Processing (NLP):
- Enables an AI Support Agent to interpret user questions, detect intent, and respond conversationally.
- Identifies key phrases and topics in real-world language, even when users don’t phrase queries perfectly.
Machine Learning:
- Learns from historical data—like past tickets, FAQs, or documentation—and continuously refines responses.
- Allows the agent to draw connections between issues and solutions, improving accuracy with each interaction.
Combining NLP and Machine Learning makes an AI Support Agent resilient and adaptive, capable of understanding new or unexpected user requests over time.

Integrations with Knowledge Bases and Ticketing Systems
For maximum efficiency, an AI Support Agent connects directly to your organization’s ticketing platform—such as Jira, Salesforce Cases, Zendesk, or ServiceNow. This integration streamlines the process of creating, updating, or escalating support requests. Additionally, linking the agent to your knowledge base (product guides, FAQs, manuals) ensures it can swiftly retrieve relevant information.
CTA (Mid-Content): Want to see how seamless these integrations can be? Create your first AI Service Desk Agent with Enjo and unify your support
Continuous Learning and Improvement
A true hallmark of a modern AI Support Agent is the ability to continuously learn. Each interaction—successful or not—feeds back into a training loop, refining the agent’s language models. Over time, your AI solution transforms into a robust, context-aware resource, capable of handling increasingly complex tickets while maintaining quality responses.
- Feedback Loops: Solicits user or agent ratings to gauge response helpfulness.
- Incremental Updates: Incorporates new product releases, policies, or FAQs into its knowledge repository automatically.
By systematically analyzing ticket outcomes and user satisfaction metrics, the AI Support Agent can identify and close knowledge gaps, ultimately boosting efficiency and user confidence in the system.
Generations of AI Support Tools
The concept of automating support has evolved significantly over time. Understanding these generations helps illustrate where modern platforms—like Enjo—fit in the broader landscape of AI customer service solutions.
Gen 1: Rule-Based Chatbots
- Rigid Script Flows
Early chatbots adhered strictly to predefined scripts and if-this-then-that rules. Any query outside their programming would confuse them. - Limited Scope
These solutions could handle only basic tasks—like providing office hours or simple FAQs—due to their lack of true language understanding. - High Maintenance
Updates required manual intervention. Admins often had to rewrite entire decision trees when new support scenarios emerged.
Gen 2: Machine Learning Chatbots
- Data-Driven Responses
With machine learning, chatbots could understand a wider range of inputs by analyzing existing ticket data or FAQs. - Improved Accuracy
Classification models and intent recognition algorithms improved response quality. However, they still struggled with complex or multi-part inquiries. - Labor-Intensive Training
While more flexible than rule-based systems, Gen 2 chatbots needed large, labeled datasets and frequent tuning to maintain high performance.
Gen 3: Generative AI Agents
- Advanced Language Models
Generative AI leverages robust NLP frameworks, enabling the agent to understand nuance and context in user queries. - Dynamic Learning
A Gen 3 AI Support Agent can explore multiple knowledge sources—like historical tickets or product documentation—to craft real-time, context-aware answers. - Proactive & Autonomous
These agents don’t just respond; they can initiate actions, such as creating tickets or updating workflows, reducing your team’s manual workload.
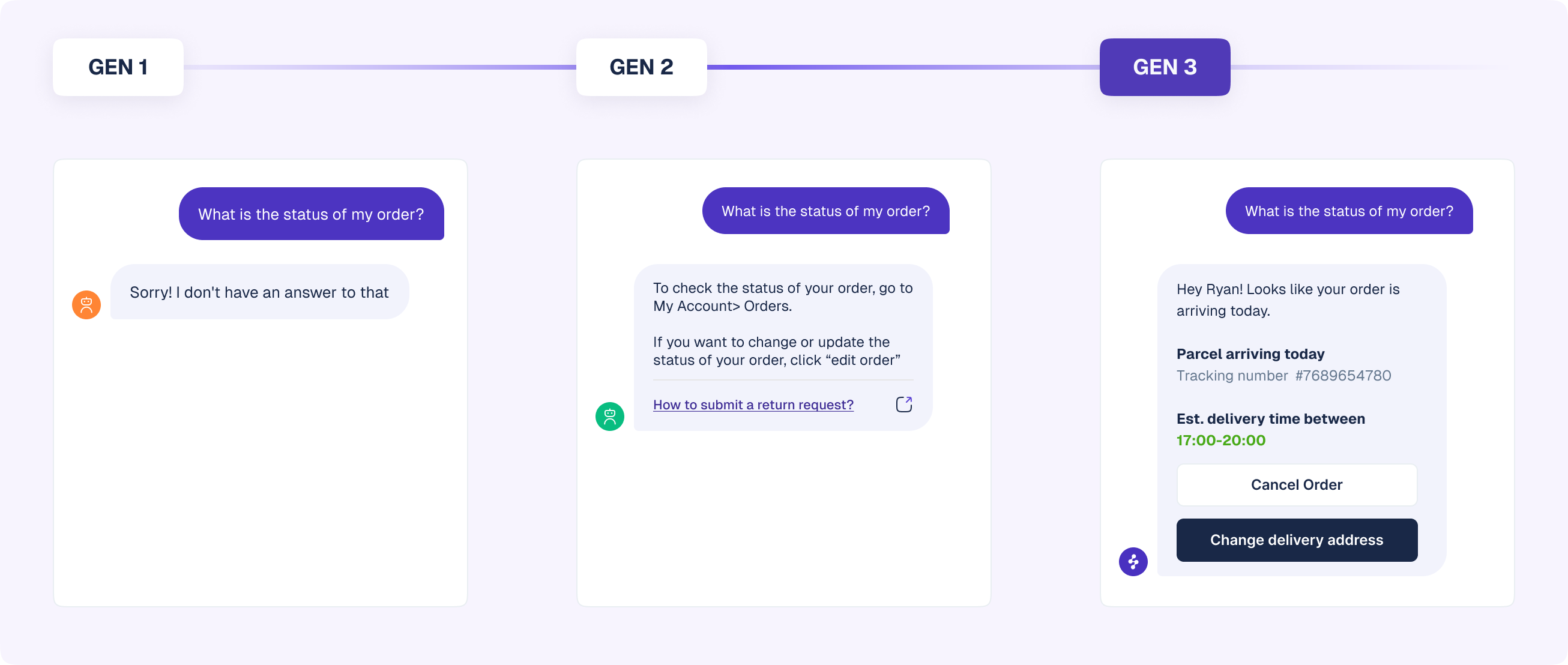
Compared to Gen 1 and Gen 2, the Gen 3 AI Support Agent stands out for its adaptability, efficiency, and deeper conversational intelligence. By adopting a solution at this level, enterprises can drastically streamline day-to-day support, enhance user satisfaction, and future-proof their service model.
Key Considerations for Implementing AI-Powered Customer Service
Before rolling out an AI Support Agent across your organisation, it’s essential to address a few foundational elements. By planning for data accuracy, security, seamless integrations, and ongoing optimization, you set your AI agents up for success from day one.
Data Preparation and Governance
An AI Support Agent relies on clean, well-structured data to generate accurate responses. This means:
- Consolidating FAQ Content and Past Tickets: Gather the most common questions, resolutions, and product documentation in a central knowledge base.
- Ensuring Data Quality: Clean up outdated entries, remove duplications, and confirm that all information is up to date.
- Implementing Access Controls: Regulate who can update or delete key articles, preventing version conflicts and misinformation.
Security and Compliance Requirements
Especially for mid to large enterprises, security and compliance are non-negotiable:
- Encryption: Ensure data is encrypted both in transit and at rest.
- Role-Based Permissions: Assign user privileges carefully so that only authorized team members can access sensitive data.
- Regulatory Standards: Verify that the AI platform meets relevant regulations (e.g., GDPR, HIPAA, SOC 2) depending on your industry.
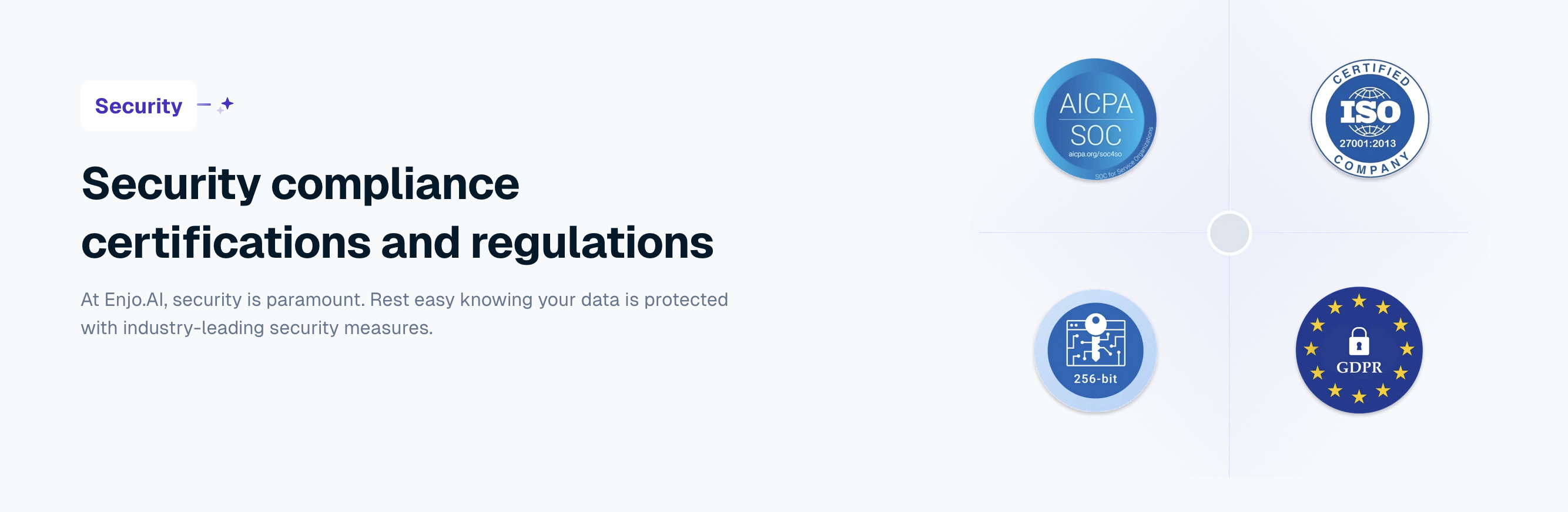
Integration Strategy with Existing Workflows
An AI Support Agent becomes far more powerful when it connects seamlessly to your existing tech stack:
- Ticketing Systems: Integrate with Jira, Salesforce Cases, Zendesk, or ServiceNow for creating and managing requests.
- Collaboration Channels: Deploy the agent in Slack or Microsoft Teams so users can chat and resolve issues in real time.
- Knowledge Repositories: Link to Confluence, SharePoint, or other document hubs where relevant content resides.
Monitoring, Analytics, and Continuous Optimization
Implementing an AI Support Agent is not a “set it and forget it” scenario. Regular evaluations and iterative improvements are crucial:
- Performance Dashboards: Track metrics like average resolution time, ticket deflection rate, and user satisfaction.
- Feedback Mechanisms: Allow users and support staff to rate the helpfulness of AI-generated responses.
- Ongoing Training: Update agent knowledge with new product information or policy changes to keep support accurate and up to date.
Top Stats and Facts About AI Support Agents
Industry Benchmarks and ROI Indicators
- Ticket Deflection Rates: Studies indicate that intelligent AI agents can deflect up to 70-80% of low-level support tickets, freeing human agents to focus on higher-complexity tasks.
- Cost Savings: By automating routine queries, enterprises often see a 30-40% reduction in operational expenses—savings that can be reinvested in strategic initiatives.
- User Satisfaction: Faster response times lead to improved CSAT scores. Organizations report boosts ranging from 10-20% after integrating AI-driven support.
- Employee Productivity: Internal teams leveraging an AI Support Agent experience less administrative burden, enabling them to handle complex issues or focus on value-added projects.
Adoption Rates and Future Projections
- Growing Demand: Gartner predicts that by 2026, almost 75% of customer service interactions will be handled by AI-driven tools.
- Industry-Wide Shift: Sectors like IT, e-commerce, and customer support operations see particularly rapid AI adoption—fueled by rising customer expectations and a need for round-the-clock service.
- Continuous Evolution: As AI language models grow more sophisticated, future AI Support Agents will begin to predict user needs before they arise, offering truly proactive and personalized services.
The Evolving Role of AI in Enterprise
The AI Support Agent is no longer a futuristic vision—it’s a practical reality reshaping support and overall workflows. Where early AI solutions focused on handling predefined tasks, today’s generative AI tools go beyond reactive support to proactively anticipate user needs.
From Reactive Support to Proactive Solutions
Traditional AI implementations responded solely to user prompts. Modern AI agents harness deep learning models, enabling them to:
- Predict User Queries: Anticipate next steps based on historical patterns.
- Preempt System Failures: Flag potential technical issues or policy gaps before they escalate.
- Offer Customized Recommendations: Tailor responses based on individual user profiles or known preferences.
By shifting to proactive engagement, the AI Support Agent helps users solve problems they might not even realize they have—ultimately boosting efficiency across the organisation.
How AI Shapes the Future of Work
Today’s workforce demands rapid communication and immediate access to resources. AI-driven solutions address these needs by:
- Streamlining Collaboration: Integrating with tools like Slack or Teams to provide on-demand insights without leaving the conversation.
- Driving Operational Excellence: Automating mundane tasks, so teams can allocate their time to strategic endeavors.
- Enhancing Knowledge Management: Continuously learning from every interaction, reducing knowledge silos across departments.
As AI evolves, we can expect new capabilities, including sentiment analysis for more empathetic responses and advanced predictive analytics for preemptive troubleshooting. By staying ahead of these trends, organizations can transform their operations, employee engagement, and customer satisfaction with a reliable AI Support Agent at the core.

